Probability and Statistics > Basic Statistics > What is Bias in Statistics?
- What is Bias in Statistics?
- Biased Estimators.
- What is Selection Bias?
- Survivorship Bias.
- Social Desirability Bias
- Acquiescence Bias
- Availability Bias
- Other Types of Bias
What is Bias in Statistics?
Bias is the tendency of a statistic to overestimate or underestimate the population parameter you’re trying to measure. For example, if your population has a mean weight of 150 pounds but your statistic gives you 100 pounds, then there may be some bias in your statistic.
Bias can seep into your results for many reasons, including sampling or measurement errors, or unrepresentative samples.
Sampling Errors
Sampling error is the tendency for a statistic not to exactly match the population. Error doesn’t necessarily mean that a mistake was made in your sampling; Sampling Variability could be a more accurate name.
For example, let’s say you have a population in the United States with an average height of 5 feet 9 inches. If you take a sample, even a fairly sizable sample of say, 10,000 people, it’s unlikely that you’ll get exactly 5 feet 9 inches. You might get very close, perhaps to within a fraction of an inch. If you repeat the experiment, you might get another very close result. For example:
- Experiment 1 = 5 feet 8.9 inches,
- Experiment 2 = 5 feet 9.1 inches.
This tendency for statistics to get very close, but not exactly right, is called sampling error.
Note: If the statistic is unbiased, the average of all statistics from all samples will average the true population parameter.
Back to Top
Measurement Errors
Measurement errors are where a provided response is different from the real value. For example, you might survey to find out if a person voted for Candidate X. A person may have voted for him, but if they are confused by the wording of the questionnaire they might mistakenly respond that they did not vote for him.
Several factors may cause measurement error, including:
- The way the interviewer poses the question.
- The wording on the questionnaire.
- The way the data is collected.
- The respondent’s record-keeping system.
Biased Estimator
Biased Estimators
An estimator is a rule for calculating an estimate of a quantity based on observed data. For example, you might have a rule to calculate a population mean. The result of using the rule is an estimate (a statistic) that hopefully is a true reflection of the population.
The bias of an estimator is the difference between the statistic’s expected value and the true value of the population parameter.
If the statistic is a true reflection of a population parameter it is an unbiased estimator. If it is not a true reflection of a population parameter it is a biased estimator.
The word bias in the regular English language implies that you have a personal reason to misrepresent a piece of information. However, in statistics, it doesn’t mean that the interviewer, the researcher or even the respondent in an interview is biased in some way. It just means that the estimator being used doesn’t produce a good estimate.
Example of a Biased Estimator
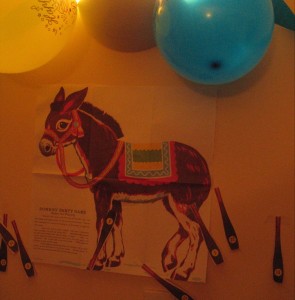
You are playing the party game “Pin the tail on the donkey.” (If you aren’t familiar with the game, a picture of a donkey is placed on the wall and you are given a paper tail to pin on the donkey while you are blindfolded. The person who pins the tail closest to the actual spot where the real tail should go wins the game). You try six times to pin the tail in the right place and each time you pin the tail in the wrong place, at the bottom or to the front of the donkey. Your estimation for the actual spot where the tail should have gone is a biased estimator because you put the tails in the wrong place.
Back to Top
What is Selection Bias in Statistics?
Ideally, you should randomly select every participant in a survey. But, sometimes biases creep in, whether intentional or unintentional. Selection bias takes away from the “randomness” you are hoping to achieve and is usually a result of not using the correct procedures to choose your participants.
Back to Top
Examples of Selection Bias Types
Healthy Worker Effect
In general, people who are working are healthier than people who are unemployed. The healthy worker effect, a type of membership bias, is a particular type of selection bias that happens when you are studying the effects of occupational exposure to a compound, like asbestos, and only include employed persons in your study. These people are less likely to suffer from the effects of exposure than people who are currently unemployed, including people who are disabled due to the exposure.
Back to Top
Hospital Patient Bias
This type of selection bias (also called Berkson’s Paradox) is when a case-control study uses hospitalized patients as controls. If those patients are hospitalized due to a connection with the disease being studied, then any measure of the drug or procedure’s effect may weaken.
Back to Top
Non-response bias
Non-response bias is a type of bias that happens when some people fail to respond to a survey. People may refuse to answer, or lack the time or inclination to answer.
For example, you may have a survey about cheating on tax returns; the people most likely to not answer are the very people you are trying to reach: cheaters on tax returns. This type of selection bias can also creep in with other types of sensitive information, like questions about prostitution, alcoholism, or illegal drug use.
Non-response bias can also become a factor if you haven’t constructed your survey properly. For example, if you have a snail mail survey for young adults or a smartphone survey for older adults; both these scenarios are likely to lead to a lower response rate for your targeted population.
Missing data can be filled in (“imputed”) with procedures like Multiple Imputation.
Undercoverage
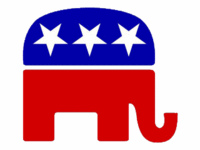
Similar to non-response bias, undercoverage is when your respondents aren’t from the population you hoped for. A classic example is the Literary digest voter survey, which predicted Franklin Roosevelt would beat by Alfred Landon in the 1936 presidential election. The survey had an undercoverage of low income voters, who were more likely to be Democrats. Ironically, the survey was one of the largest and most expensive surveys undertaken, with a sample of about 2.4 million people; despite this huge number of people, the sampling error was a massive 19 percent.
Back to Top
Voluntary response bias in statistics
Some surveys — like call in radio shows — tend to attract very opinionated people. These types of voluntary responses lead to an under-representation of the general population in favor of strong opinions.
Back to Top
Volunteer Bias
This crops up frequently in clinical trials; the people who volunteer for the trials may not represent the population you are trying to target. For example, if your study is for a new drug to treat diabetes and you offer significant compensation, people with low socio-economic background may make up the bulk of volunteers.
Back to Top
Survivorship Bias
Survivorship bias is a type of selection bias, which results in a sample that isn’t reflective of the actual population. With survivorship bias, you concentrate on the “survivors” of a particular process. The concept sounds simple, but in reality it’s tricky to implement.
Examples of Survivorship Bias in Statistics
Take a simple example. The probability of getting a full house in poker is 0.001441, or about .01%. But the odds of getting a full house after all of the cards have been dealt is 1. Imagine for a moment that you know nothing about card games (in real life, we often know little about the phenomenon we’re studying). If you were studying “full house in poker” and only looked at the survivors (the successes). You would conclude, erroneously, that the probability of getting a full house is 1 because you didn’t take into account all of the failures.
In Business
Want to succeed in business? Create a Fortune 100 company? Be the next Bill Gates? If mass-market paperbacks are all true, all you have to do is study how businesses are successful. Some New York Times best sellers on this topic include:
- Outliers by Malcolm Gladwell. Why some people succeed.
- Steve Jobs by Walter Isaacson. A biography of the Entrepreneur.
- Various other “How to be a business success” stories.
The fact is success is more likely if you study failures — those businesses that dropped out along the way — rather than successes. The success stories are few and far between and have a lot to do with luck in addition to business know how and a good product. Want to become successful like Steve Jobs? Dropping out of college, lying to get your first job, and experimenting with psychedelics probably have little to do with it.
Abraham Wald’s Naval Work
In World War II, statistician Abraham Wald tried to determine how to minimize bomber losses. Prior to Wald’s work, researchers from the Center for Naval Analysis analyzed bombers that came back with damage and recommended reinforcement of damaged areas on all bombers. However, they didn’t take into account that only the surviving aircraft came back; the bombers that did not survive likely had damage to other, more critical areas. Wald recognized that survivorship bias played a part in the Center for Naval Analysis’s decision and recommended basically the opposite–the reinforcement of areas that had not been hit on the surviving aircraft. You can find an article about Wald’s work here.
Social Desirability Bias
Social desirability bias is the tendency to answer questionnaires or surveys according to what is socially acceptable. People tend to report inaccurately on sensitive topics like abortion, drug use, or prostitution. This is usually attributed to embarrassment or lack of comfort in revealing true feelings or attitudes.
Indirect questions (i.e. general, non personal questions) are advisable when dealing with sensitive issues as they tend to make people more honest about their true feelings.
Forced choice items and use of proxy subjects can also reduce or prevent this type of bias.
Acquiescence Bias in Statistics
This type of bias usually happens because people want to be polite or to be agreeable, although it can also happen because people want to skim through a survey quickly. See: Acquiescence Bias.
Availability Bias in Statistics
This type of bias is where you make a probability calculation based on the first thing that comes to mind. Advertisers use it to their advantage. See: Availability Bias.
Other Types of Bias in Statistics
- Accidental Bias
- Aggregation Bias
- Allocation Bias
- Ascertainment Bias
- Assignment Bias
- Attrition Bias.
- Central Tendency Bias.
- Diagnostic Bias
- Funding Bias
- Information Bias
- Misclassification Bias
- Neyman Bias
- Observer Bias
- Performance Bias
- Publication Bias
- Referral Bias
- Reporting Bias
- Self-selection bias
- Spectrum Bias
- Verification Bias
- Wording Bias