Statistics Definitions > Internal Validity
What is Internal Validity?
Note: If you aren’t familiar with confounding, read this article first:
Confounding Variables
Internal validity is a way to measure if research is sound (i.e. was the research done right?). It is related to how many confounding variables you have in your experiment. If you run an experiment and avoid confounding variables, your internal validity is high; the more confounding you have, the lower your internal validity. In a perfect world, your experiment would have a high internal validity. This would allow you to have high confidence that the results of your experiment are caused by only one independent variable.
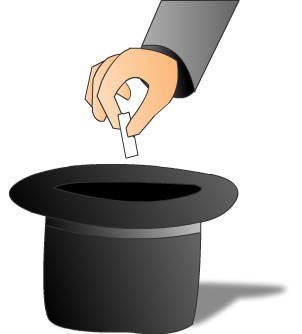
For example, let’s suppose you ran an experiment to see if mice lost weight when they exercised on a wheel. You used good experimental practices, like random samples, and you used control variables to account for other things that might cause weight loss (change in diet, disease, age etc.). In other words, you accounted for the confounding variables that might affect your data and your experiment has high validity.
On the other hand, if you failed to use random sampling or control variables at all, your risk of confounding is extremely high. Therefore your internal validity would be very low.
External vs. Internal Validity
Internal validity is a way to gauge how strong your research methods were. External validity helps to answer the question: can the research be applied to the “real world”? If your research is applicable to other situations, external validity is high. If the research cannot be replicated in other situations, external validity is low.
Things that can Affect Validity
Sometimes, confounding variables may not be that obvious. The list of usual suspects for things that can have an effect on internal validity is long. It includes:
- Regression to the mean. This means that subjects in the experiment with extreme scores will tend to move towards the average.
- Pre-testing subjects. This may have unexpected consequences as it may be impossible to tell how the pre-test and during-tests interact. If “logical reasoning” is your dependent variable, participants may get clues from the pre-test.
- Changing the instruments during the study.
- Participants dropping out of the study. This is usually a bigger threat for experimental designs with more than one group.
- Failure to complete protocols.
- Something unexpected changes during the experiment, affecting the dependent variable.
References
Dodge, Y. (2008). The Concise Encyclopedia of Statistics. Springer.
Everitt, B. S.; Skrondal, A. (2010), The Cambridge Dictionary of Statistics, Cambridge University Press.
Vogt, W.P. (2005). Dictionary of Statistics & Methodology: A Nontechnical Guide for the Social Sciences. SAGE.
Wheelan, C. (2014). Naked Statistics. W. W. Norton & Company